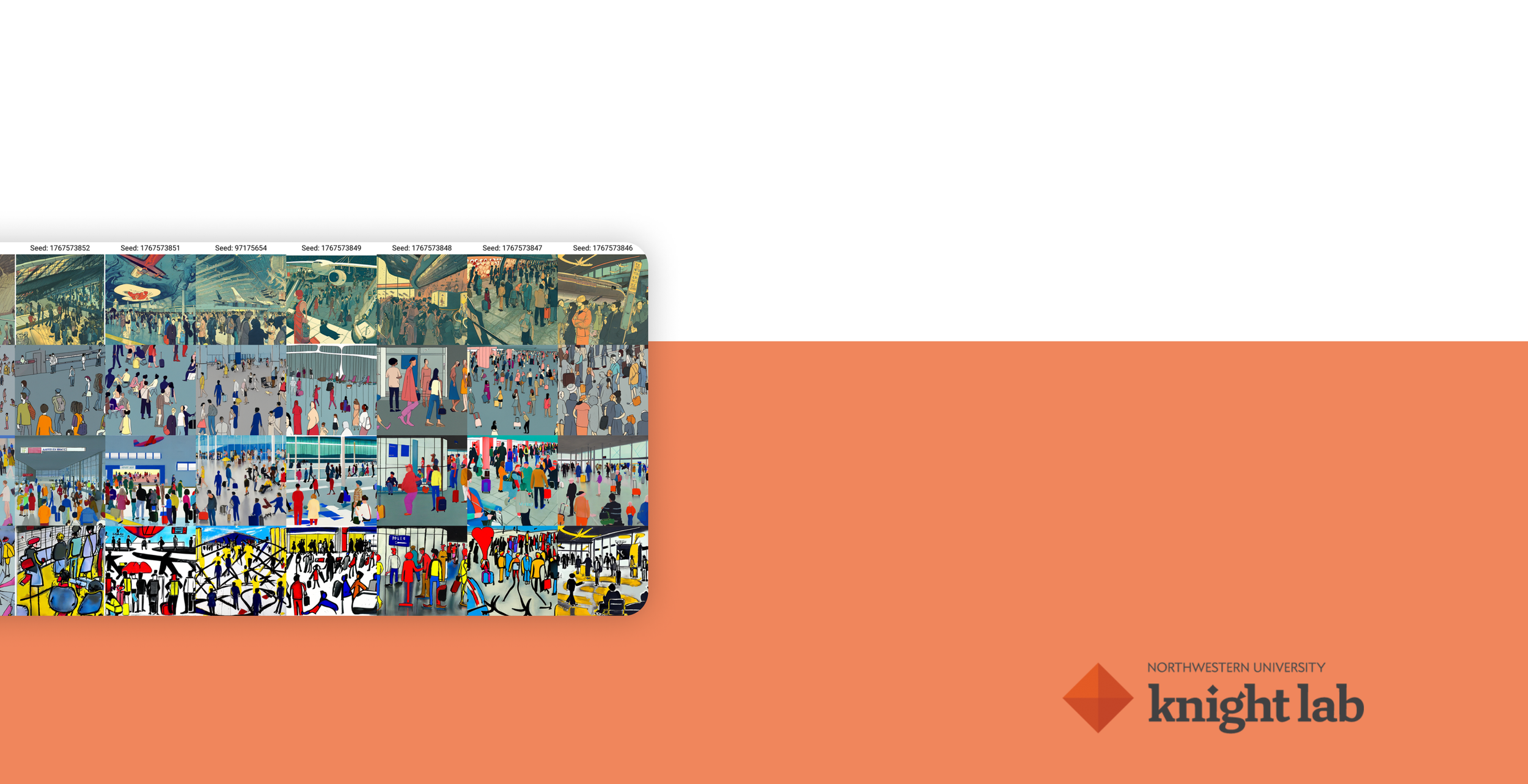
AI & Diversity of Editorial Art
Low-cost art generation by AI is ideal to generate editorial visuals for low-budget newsrooms and individual journalists.However, it’s extremely racially and sexually discriminative.
Within 10 weeks, I led the AI Art training project at Knight Lab, a media innovation lab in NU, aiming to enable AI Art model to produce editorial visuals with diverse perspectives.
ROLE AI Art Lead Researcher
TEAM Me and five other AI Art researchers
TIME 10 weeks
1 Why AI Art Generation Model Needs Training?
Currently, the AI Art generation model, taking the example of Stable Diffusion has strong racial and sexual bias.
This is because it was mainly trained mainly by White male artists. Therefore, they often represent this group’s perspectives.
For example, under a prompt for generating anxious teenager images based on a news article Idaho Murders Suspect Felt ‘No Emotion’ and ‘Little Remorse’ as a Teen, most AI-generated visuals demonstrate a gloomy female:
In another news article, Google axes 12,000 jobs as layoffs spread across tech sector, AI depicted most laid-off employees as people of color:
Besides, AI-generated visuals by default are in a realistic photo style. Even after adding “illustration” as a keyword, the visuals are not editorial enough for journalistic purposes.
2 Train Stable Diffusion with Visuals from Non-White Female Illustrators
To alleviate AI’s racial and sexual bias and make it generate visuals with editorial standards, we started to train Stable Diffusion, one of the most popular text-to-image models with a visual database of non-White female illustrator’s works.
The training process involves four steps:
Firstly, select five articles on different topics and create basic prompts based on them. Created prompts per the gist of the articles. The keyword “illustration” is added to the end of each prompt.
Secondly, we made table Diffusion generate two control groups.
Taking the news article Flight Disruptions Ease as FAA Says Operations Are Back to Normal After Outage as an example, control group one (C1) consists of visuals based on the prompt “many people, airport, stranded, waiting, chaos, illustration” without the influence of artists:
Control group two (C2) consists of visuals based on the prompts with the influence of trained artists:
Thirdly, train Stable Diffusion via Text Inversion. In total, we selected 20 female artists of diverse backgrounds from all over the world. They are active in producing visuals for news reporting platforms, such as New York Times and Wall Street Journals.
Finally, after the training, Stable Diffusion generates the test group. It consists of visuals based on the same prompts with the influence of newly-trained artists.
Below are the Airport Chaos visuals generated by Stable Diffusion with the influence of Eiko Ojala:
The training is tested effective. ✅
3 Train SD based on Editorial Genres
Training the model based on editorial illustrators was not ideal since it’s under too much personal influence and has the risk of copyright infringement. We changed another way of training SD based on editorial genres - editorial symbolism and editorial collage.
For each genre, we established a database of around 150 artworks from 15 non-white female illustrators and trained the SD as the tags of “EditorialCollage” or “EditorialSymbolic”, instead of artists’ names.
Here is the result of AI visuals for Twinkle, twinkle fading stars: Hiding in our brighter skies. It combines the visuals before training, trained groups with the influence of editorial collage, editorial scene, and editorial symbolic:
👆
Prompt: Star-filled sky with visible light pollution and a child gazing up at the stars, illustration, concept art, + test variables
4 Training SD with Hypernetworks
After training, Stable Diffusion understands editorial genres and could represent a more diverse perspective.
However, when using the training methods of Text Inversion, we have to create new concept tags. The visual effects of these tags are not stable enough since training a tag/concept requires thousands of visuals.
we found another training method, Hypernetworks. that can better fit the training data with pre-existing concepts.
This means that this method could make the model grow over time by updating the concept. What’s more, it can make the model generate photos with previous learning, thus leading to higher-resolution results.
Therefore, with the same editorial database, we trained SD with Hypernetworks and acquired ideal results.
👆
Result after training SD with Hypernetworks
Prompt: Star-filled sky with visible light pollution and a child gazing up at the stars, illustration, concept art, EditorialSymbolic
5 Towards a Diverse AI Era: Utilize AI in Visual Storytelling
This project pioneers a more inclusive AI-generated visual world. Previously, AI Art was not proper in mass media because of its bias. After including more diverse training databases, I believe we could step into a diverse era where AI Art generation is able to benefit visual storytelling.
In the end, here is a video story made with AI-generated visuals to showcase its potential in engaging storytelling. News article: Twinkle, twinkle fading stars: Hiding in our brighter skies.
Let’s welcome the diverse AI era.
Any Thoughts?
Thank you for reading! Please don’t hesitate to email me at irisyingjieliu@gmail.com, if you have any feedback.
I appreciate your precious advice! ❤️
You might be interested in…
Peppermint
Usability Testing & Dashboard Redesign
OURA Ring
UX Design & Content Strategy
Purring
UX Design & Research